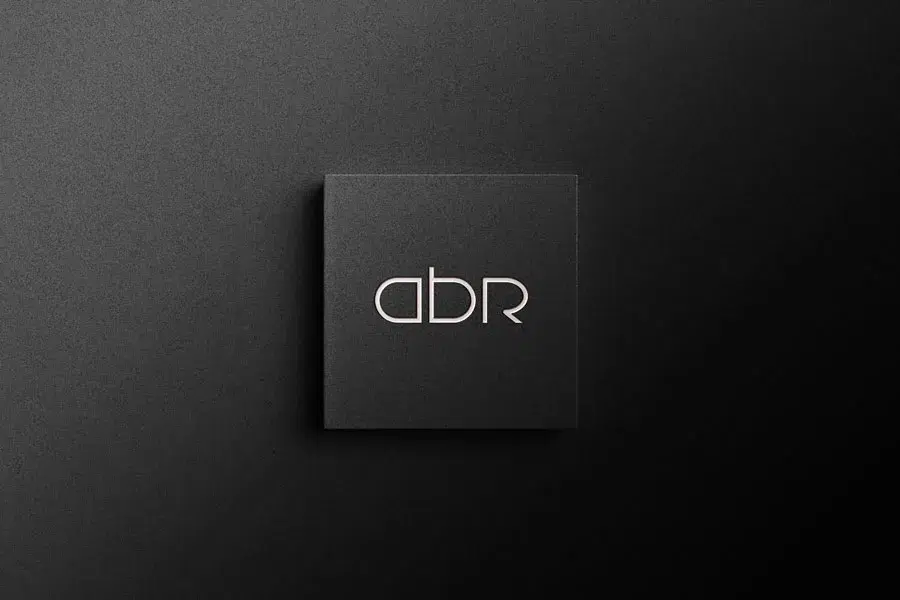
ABR demonstrates the world’s first single chip solution for full vocabulary speech recognition
SAN JOSE, CA, [Sep 9] – Applied Brain Research (ABR), a leader in the development of AI solutions, is demonstrating the world’s first self-contained single-chip